Harnessing Healthcare Datasets for Machine Learning: A Transformative Approach
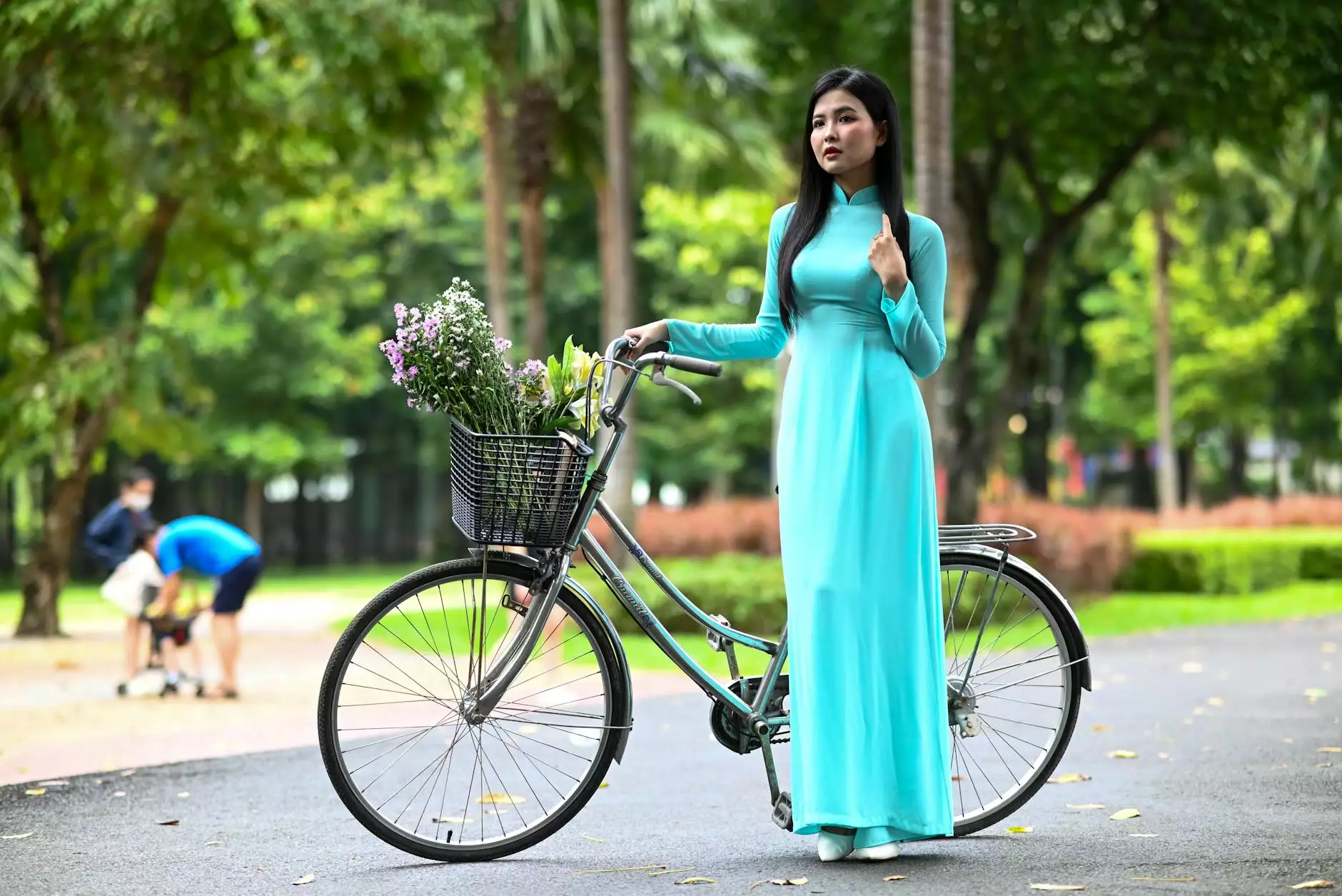
In the ever-evolving landscape of healthcare, the integration of machine learning has emerged as a pivotal force driving transformation. As a fundamental component of this evolution, healthcare datasets for machine learning are vital, unlocking unprecedented opportunities in patient care, operational efficiency, and business development. This article delves deep into how healthcare datasets fuel machine learning applications, their significance for businesses like Keymakr, and the future implications for the industry.
The Significance of Healthcare Datasets in Machine Learning
Healthcare datasets are collections of information that include patient records, treatment outcomes, and medical imaging data, among other information types. When applied to machine learning, these datasets enable algorithms to identify patterns, predict outcomes, and inform decision-making processes. The significance of utilizing these datasets lies in their ability to:
- Enhance Predictive Analytics: By analyzing historical data, machine learning models can predict future health outcomes and facilitate early intervention.
- Improve Patient Care: Personalized treatment plans can be developed by understanding patient history and response to therapies.
- Streamline Operations: Machine learning can identify inefficiencies within healthcare systems, optimizing resource allocation and reducing operational costs.
- Support Research and Development: Accelerated drug discovery and clinical trials benefit immensely from the insights derived from these datasets.
Understanding Healthcare Datasets for Machine Learning
Healthcare datasets for machine learning can be categorized into several types, each serving unique purposes and contributing differently to the overall healthcare landscape:
1. Electronic Health Records (EHRs)
EHRs are comprehensive digital versions of patients' paper charts and contain key information such as medical history, diagnoses, medications, treatment plans, and immunization dates. The structured nature of this data enables effective training of machine learning models.
2. Medical Imaging Data
Medical imaging datasets, including X-rays, MRIs, and CT scans, are critical for developing machine learning algorithms capable of analyzing visual data. For instance, convolutional neural networks (CNNs) can be employed to detect anomalies in images.
3. Genomic Data
Genomic datasets contain information about individual genetic profiles and mutational landscapes. Machine learning applications in genomics can lead to the identification of disease predispositions and effective precision medicine strategies.
4. Wearable Device Data
Data collected from wearable devices, such as smartwatches and health monitors, provide real-time health insights. Machine learning can utilize this data for synchronous monitoring and proactive health management.
The Role of Machine Learning in Business: Keymakr's Perspective
For businesses like Keymakr, which operate within the domains of home services, keys, and locksmiths, the integration of healthcare datasets and machine learning can offer numerous competitive advantages. Keymakr emphasizes technology adoption for providing top-notch service. Here's how:
1. Enhancing Customer Service
By analyzing customer data, Keymakr can tailor its offerings to better suit the needs of its clientele. For instance, understanding seasonal demand fluctuations can help optimize staffing and service availability.
2. Predictive Maintenance of Tools
Utilizing machine learning algorithms on equipment performance data, Keymakr can predict maintenance needs for locksmith tools and shared property access systems, preventing service disruptions and minimizing downtime.
3. Improved Marketing Strategies
Machine learning provides insights into customer behavior, allowing for more effective targeting and personalized marketing. By analyzing past service requests, Keymakr can anticipate customer needs and deliver tailored promotions, ultimately fostering increased customer retention.
4. Streamlining Operations
Using machine learning to analyze operational data can lead to process improvements. For instance, automating scheduling and logistics can enhance service efficiency and customer satisfaction.
Data Privacy and Ethical Considerations
With great power comes great responsibility; hence, leveraging healthcare datasets for machine learning in the context of business must be approached with caution. Ethical considerations and data privacy are paramount in ensuring that advancements do not come at the cost of patient trust and safety.
1. Compliance with Regulations
Businesses using healthcare datasets must adhere to regulations such as HIPAA (Health Insurance Portability and Accountability Act) in the United States. This ensures that patient data is handled securely and ethically, safeguarding sensitive information.
2. Bias in Machine Learning Models
Algorithmic bias can lead to disparities in healthcare outcomes. It is crucial for businesses to continually monitor their machine learning models and datasets for bias, ensuring equitable treatment across diverse populations.
Future Trends in Healthcare Datasets and Machine Learning
As we look to the future, the integration of healthcare datasets for machine learning is set to evolve significantly. Here are some anticipated trends:
1. Enhanced Interoperability
Efforts to improve the interoperability of healthcare systems will facilitate the seamless exchange of data, ultimately enriching the datasets available for machine learning applications.
2. Real-time Data Utilization
With advancements in technology, real-time data collection will become more prevalent, providing more immediate insights for machine learning applications. This will allow businesses to respond proactively to changes in healthcare demands.
3. Increased Adoption of AI in Telehealth
The rise of telehealth services presents a rich opportunity for leveraging healthcare datasets. Machine learning can enhance virtual interactions, making remote diagnostics more efficient and personalized.
4. Focus on Patient-Centric Solutions
Future developments in machine learning will likely prioritize patient outcomes. By focusing on patient-centered care, healthcare providers and associated businesses can leverage analytics for improved service offerings.
Conclusion
The power of healthcare datasets for machine learning cannot be overstated. For businesses like Keymakr, tapping into this resource can lead to remarkable improvements in service delivery, operational efficiency, and customer satisfaction. As the healthcare landscape continues to evolve, the strategic integration of machine learning will be crucial in navigating challenges and seizing opportunities for growth. The future is bright for those willing to embrace the transformative capabilities of data-driven insights.
Call to Action
If you are part of the healthcare or business community and want to explore how to leverage healthcare datasets for machine learning effectively, consider partnering with experts in the field. Together, we can pave the way for innovation and excellence in service delivery.